Elad Harel
Research
Deep Spectroscopy for Breakthrough Molecular Discovery
(Research Description PDF)
Research in the Harel lab is aimed at developing nonlinear spectroscopy and imaging methods towards a deeper understanding of molecular systems and in discovering new applications of these techniques to real-world problems. On the fundamental side, the group is attempting to understand how factors at the molecular scale influence macroscopic observables in complex and dynamical systems. These local changes may be, for instance, disruptions in the intramolecular forces that hold a protein in its folded state, weak lattice phonons that scatter charge carriers in semiconductors, or light-induced electron transfer in photosynthetic organisms. A fundamental understanding of the connection between such microscopic interactions and their influence on macroscopic system properties in complex, condensed- or solidphase environments is critical to informing the designs of next-generation catalysts, new small-molecule drugs, and novel optical materials with tailored functionality. However, as the systems becoming increasingly complex, understanding the myriad of factors that dictate function of a molecule or performance of a system becomes too convoluted to decipher by conventional approaches. This is one of the main reasons that breakthrough molecular and materials discoveries are largely serendipitous. A big data approach to these challenges could quickly inform on the cause and effect of chemical and physical changes across a vast phase space that is too large to search systematically even with the help of state-of-the-art computational resources. Deep learning approaches which have shown tremendous success in a wide range of fields from machine vision, gaming, defense, and health care to name a few, could lead to breakthrough discoveries, but only if high-quality and a sufficient volume of data was readily available. Our lab is developing tools to generate big data by combining high-throughput synthesis, rapid nonlinear optical characterization, and deep-learning based modeling. Recent efforts have been aimed at combining high-resolution scanning probe microscopy for morphology analysis with ultrafast coherent spectroscopy (e.g. pump-probe, 2D electronic, 2D Raman, and 3D/4D electronic-Raman) to gain insight into the chemical composition and electron dynamics at the nanometer length scale. Using high-throughput robotics, our lab rapidly synthesizes and analyze thousands of different solution-processed materials in a fraction of the time of conventional approaches using extremely small volumes (< 1 nL) of material. By combining ensemble and singlemolecule approaches, our approach gathers correlated data across vast regions of time, energy, and space which inform on critical factors that dictate the function and performance of complete systems rather than just the compounds that compose them. Current molecular targets include conjugated polymers used in organic photovoltaics, singlet fission materials, light-harvesting pigmentprotein complexes, colloidal quantum dots, and two- and three-dimensional halide perovskite materials used in lighting, detectors, and photovoltaic applications. A heavy emphasis of the research effort is in developing high-resolution characterization methods at the ultimate limit of detection — a single molecule or nanocrystal — where size, shape, and environment can strongly influence the chemical, optical, and physical properties; these properties are oftentimes masked by ensemble measurements. Applications of these methods include standoff detection of biomolecules with high sensitivity and specificity, chemically-specific hyperspectral imaging, super-resolution live-cell microscopy, and real-time analysis of on-demand drug production, to name a few.
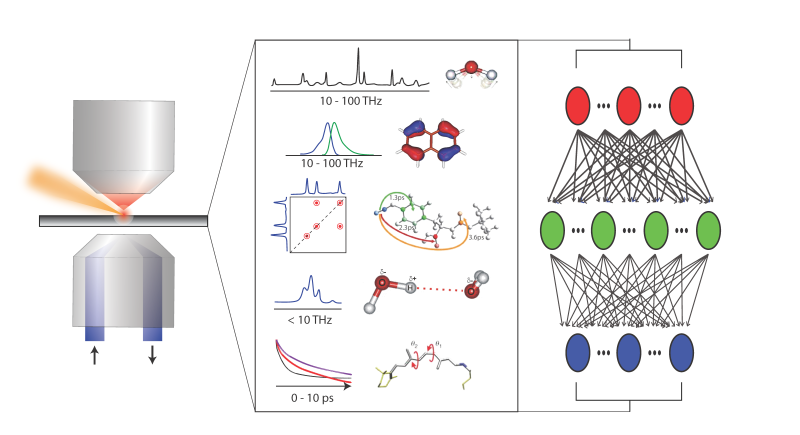
pump-probe, TR-PL, etc.) is used to generate high-quality data for deep learning.
Contact / Webpage
Area(s) of Interest
Physical (Ph)
Analytical (An)
Biological (Bi)
Material (Ma)
Theoretical and Computational (Th)
Selected Publications
Electronic Coherence Lifetimes of the Fenna-Matthews-Olson Complex and Light Harvesting II, S. Irgen-Gioro, K. Gururangan, R. G. Saer, R. E. Blankenship, E. Harel, Chem. Sci. 2019, 10.1039/C9SC03501J.
Transient Sub-Band-Gap States at Grain Boundaries of CH3NH3PbI3 Perovskite Act as Fast Temperature Relaxation Centers, X. Jiang, J. Hoffman, C. C. Stoumpos, M. G. Kanatzidis, E. Harel, ACS Energy Lett. 2019, 4, 1741-1747.
Four-Dimensional Spectroscopy of Complex Molecular Systems in Solution, A. P. Spencer, E. Harel, J. Phys. Chem. C 2019, 123, 6303 (cover article).
Coherences of Bacteriochlorophyll a Uncovered Using 3D-Electronic Spectroscopy, S. Irgen-Gioro, A. P. Spencer, W. O. Hutson, E. Harel, J. Phys. Chem. Lett. 2018, 9, 6077-6081.
Exciton-Phonon Spectroscopy of Quantum Dots Below the Single-Particle Homogeneous Linewidth, A.P. Spencer, W.O. Hutson, S. Irgen-Gioro, E. Harel, J. Phys. Chem. Lett. 2018, 9, 1503-1508.
Transient Sub-bandgap States in Halide Perovskite Thin Films, S. Nah, B. M. Spokoyny, X. Jiang, C. M. M. Soe, C. C. Stoumpos, M. G. Kanatzidis, E. Harel, Nano Lett. 2018, 18, 827-831.
Ultrafast Imaging of Carrier Cooling in Metal Halide Perovskite Thin Films, S. Nah, B. M. Spokoyny, C. M. M. Soe, C. C. Stoumpos, M. G. Kanatzidis, E. Harel, Nano Lett., 2018, 18, 1044-1048.
Spatially segregated free-carrier and exciton populations in individual lead halide perovskite grains, S. Nah, B. Spokoyny, C. Stoumpos, C. M. M. Soe, M. Kanatzidis, and E. Harel, Nat. Photonics 2017, 11, 285-289.
Quantum coherence selective 2D Raman-2D electronic spectroscopy, A.P. Spencer, W.O. Hutson, and E. Harel, Nat. Commun. 2017, 8, 14732. (highlighted as a Perspective in Science)
Mapping Multidimensional electronic structure and ultrafast dynamics with single- element detection and compressive sensing, A.P. Spencer, B. Spokoyny, S. Ray, F. Sarvari, and E. Harel, Nat. Commun. 2016, 7, 10434.
CV
B.S., B.A., 2003, Univ. of California, San Diego
Ph.D., 2008, Univ. of California, Berkeley
Postdoctoral Fellow 2008-11, University of Chicago.